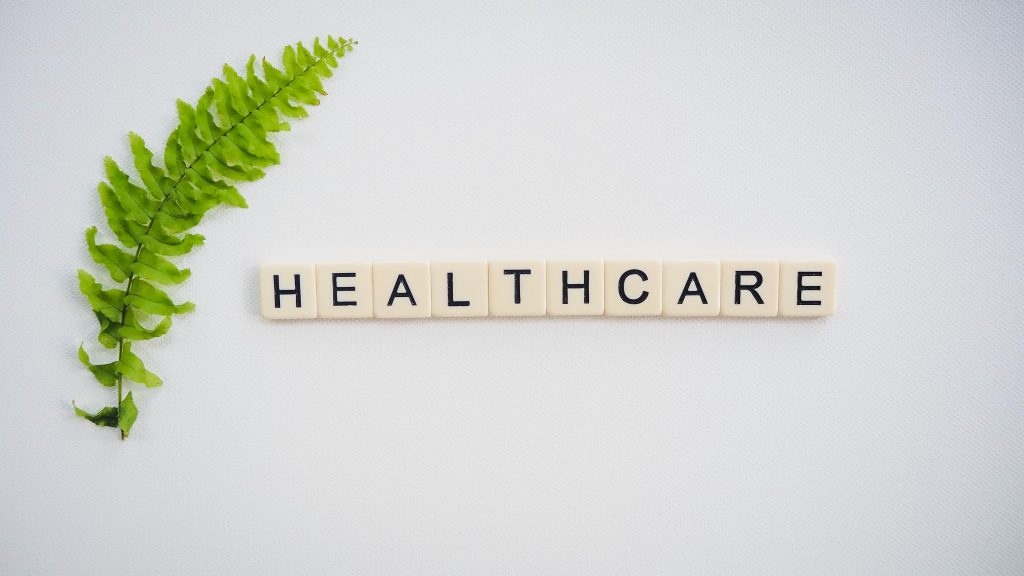
Artificial Intelligence In Healthcare
AI is transforming Healthcare, and many medical fields and specialities are starting to employ it. By utilising data patterns to make informed medical choices, AI, machine learning (ML), natural language processing (NLP), and deep understanding (DL) assist stakeholders and experts in the healthcare industry in identifying healthcare problems and solutions more quickly and accurately.
What is AI in Healthcare?
Applying ML algorithms and other cognitive technologies in medical situations falls within the broad category of AI in Healthcare. Computers and robots can mimic human cognition, learn reason, and make decisions or take action, so this is the most straightforward artificial intelligence (AI). AI in Healthcare uses computers to analyse and respond to medical data, usually to predict a specific outcome.
How is AI important in Healthcare?
Healthcare is one of the most crucial topics in the more extraordinarily extensive data landscape due to its essential role in a prosperous, healthy society. Utilising AI to analyse healthcare data has the potential to make a life or death decision. AI can assist doctors, nurses, and other healthcare workers with routine chores. AI in Healthcare may lead to better patient outcomes, better preventative care and quality of life, more precise diagnosis and treatment strategies, and better preventive care. AI can also predict and monitor the spread of harmful diseases by analysing data from the public sector and the healthcare industry. To battle diseases and pandemics, AI has the potential to play a significant role in global public health.
How can AI be used in Healthcare?
Here are the top 7 best ways in which AI can be used in Healthcare –
1. Decreasing the cost of developing medicines
Based on databases of chemical structures, supercomputers have been used to predict which potential drugs would and would not be helpful for various conditions. Convolutional neural networks, a technique similar to that used to create self-driving cars, were employed by AtomNet to decipher signals from millions of experimental data and thousands of protein structures to predict the binding of small molecules to proteins.
The cost of producing medications was reduced by convolutional neural networks’ ability to choose a safe and effective treatment option from the database looked at.
2. Analysing the unstructured data
Clinical professionals typically struggle to keep up with the most recent medical advancements while providing high-quality patient-centred care due to the enormous amounts of health data and medical records. ML technology may quickly evaluate medical units and professionals’ EHRs and biological data to provide clinicians with immediate, reliable responses.
Patient’s health information and medical records are frequently stored as complex unstructured data, making them challenging to understand and access. AI can find, collect, store, and standardise medical data regardless of format, helping with repetitive tasks and assisting clinicians with quick, accurate, tailored treatment plans and medications for their patients. This relieves clinicians from the burden of searching, identifying, collecting, and transcribing the solutions they need from piles of paper formatted EHRs.
3. Building complex platforms for drug discovery
AI algorithms can discover innovative therapeutic applications while determining their danger potential and modes of action. This process was the foundation for a drug discovery platform that enabled the company to recycle current drugs and bioactive compounds.
Combining the best aspects of biology, data science, and chemistry with automation and the most recent advancements in AI, the platform’s founding business can produce over 80 terabytes of biological data analysed by AI tools across 1.5 million weekly trials.
4. Forecasting Kidney diseases
Acute kidney injury (AKI) can be challenging for doctors to identify, but it can cause patients’ conditions to deteriorate rapidly and endanger their lives. Early diagnosis and treatment of these cases can significantly reduce the need for lifelong care and the cost of renal dialysis because it is estimated that failure to identify and treat patients accounts for 11% of hospital mortality.
Machine learning (ML) technology was created in 2019 by DeepMind Health and the Department of Veterans Affairs (VA) that can forecast AKI up to 48 hours in advance. Compared to standard treatment methods, the AI approach recognised more than 90% of patients with acute AKI 48 hours sooner.
5. Contributing to Cancer Treatment
In other cases, radiation therapy may not have a computerised database to collect and maintain EHRs, which makes cancer research and treatment difficult. Oncora Medical created a platform to aid physicians in selecting the best radiation therapy for cancer patients. The platform gathers pertinent medical information from patients, assesses the standard of care, optimises treatments, and offers specific cancer results, data, and imaging.
EHRs and automated clinical note generation decreased clinicians’ time reviewing patient records, enhancing patient care and health outcomes.
6. Accelerating the discovery of genetic medicines
AI is also successfully used to speed up the discovery and development of pharmaceuticals. Genetic diseases are encouraged by alterations in molecular phenotypes such as protein binding. Predicting the advent of genetic diseases is necessary to foresee these changes. This is possible by compiling data on all substances that have been identified as well as biomarkers pertinent to specific clinical investigations.
Deep Genomics’ AI system, for instance, handles this data. The company creates its own proprietary AI and uses it to discover cutting-edge methods for reversing the consequences of genetic mutations and to provide individualised medications for people with rare Mendelian and complex illnesses.
7. Assisting medical staff
After a sudden heart attack, the period between a 911 call and the arrival of an ambulance is crucial for recovery. For patients to have a greater chance of survival, emergency dispatchers must be able to recognise the signs of a cardiac arrest. AI may make a diagnosis at a distance by evaluating both verbal and nonverbal cues.
Final words
AI adoption in Healthcare is still hampered by issues, including the requirement to cater to specific demands and a lack of confidence in the results of machine learning systems. However, healthcare stakeholders have benefited in several ways from the application of AI in health.
By enhancing workflows and operations, aiding medical and non-medical personnel with repetitive duties, assisting users in obtaining faster answers to enquiries, and generating novel treatments and therapies, the application of AI in Healthcare may benefit patients, payers, researchers, and doctors equally.